The rapid growth in the usage of smartphones and other connected mobile devices has caused a spurt in the amount of data that flows through telecom operator networks. The operators must process, store, and extract information from the available data. By helping to optimize network use and services, improve customer experience, and improve security, Big Data analytics will help them increase productivity.
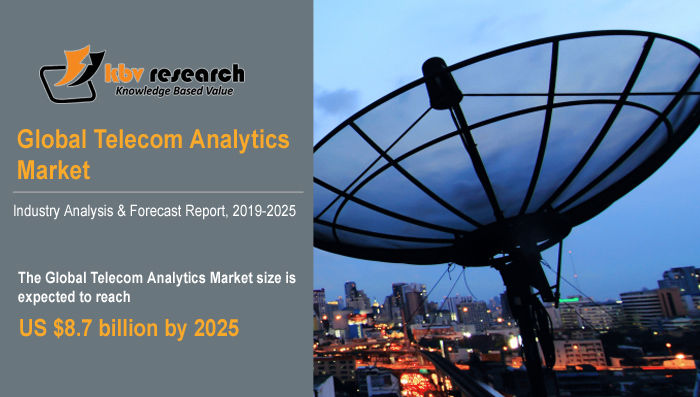
Research has shown that telecom companies have significant potential to benefit from Big Data analytics. However, Big Data's potential presents a challenge as to how does a company leverage data to raise revenues and income around the value chain, covering network operations, product creation, marketing, distribution, and customer support.
What is telecom analytics?
Telecom analytics is a form of explicitly applied and packaged business intelligence to meet the diverse needs of telecommunications organizations. The goal of telecom analytics is to lower operating costs and increase revenues by increasing revenue, reducing fraud and enhancing risk management. Telecom analytics solutions typically go far beyond what traditional business intelligence tools provide for monitoring and reporting, and can include sophisticated multidimensional analysis and forecasting.
Telecom analytics has a diverse range of applications:
Network management
The reliability of the network remains a vital aspect of performance for communications service providers. Downtime seconds can lead to millions of missed sales, increases in customer service calls and the need for fast diagnosis and remediation. IT workers need to have a network management system to navigate with so many unknowns.
When increasing amounts of rich content are being transmitted between devices, applications, and users, capacity demands on service providers have continued to increase in size and scope. Driven by convergence, M2M and the Internet of Things (IoT), both the complexity of distributed networks and the traffic rates continue to increase. Network providers provide some of the biggest, most diverse networks, serving geographically scattered facilities-from core to connection, head-end to outdoor facility, wired to wireless, and satellite. IT and network staff must provide remote access to these essential business infrastructures under all circumstances.
Customer management
The telecom industry is undergoing an explosion of competition and a period of hyper consumerization. The most immediate impact was a rise in consumer turnover. As a result, telecom companies are spending more on customer acquisition and maintenance. Nonetheless, the price point of delivering services to those consumers has remained the same, resulting in declining revenues. It's a tricky situation because consumer demand for multi-play services — such as broadband, cable TV, IP TV, mobile wallets, data, and content — can potentially improve sales if consumers can be kept.
The solution to both problems, consumer retention and improved Average User Revenue (ARPU), is the same: delivering outstanding Customer Experience (CX). Although in the last decade or so the industry has had a positive "customer first" management approach, customer expectations and perceptions are rapidly evolving. Operators need to take major technical steps to keep pace. Thankfully, the incentives to develop CX have also expanded with the rise in touchpoints.
Workforce management
Telecom workforce management is transforming as the so-called "gig economy" begins to enter the market. When operators focus more on raising their costs of building network infrastructure, an increasingly "hybrid" contract labor force and full-time workers are emerging. More and more telecommunications companies are taking a much more aggressive mixed workforce approach to have small-cell technology research done in the field and also within the conventional telecom sector work that involves boots on the ground.
Despite more emphasis on small cell installations and revenues that do not keep up with the mobile data traffic boom, operators are looking to cut labor costs. Technologies related to delivery are also keeping pace. Test vendors, for example, have spent the last few years introducing onto the market more instruments that have integrated capabilities and are often built for ease of use so that field techs can do some of the research that engineers used to do.
Role of big data in telecom analytics
Improving customer experience
The key use case for Big Data and telecom predictive analytics is optimizing customer service. For example, telecommunications marketing departments use clustering and unsupervised machine learning to segment the audience and then target individual clients.
Developing a library of more than 50 offers and then setting up a system to easily launch and evaluate relevant initiatives, telecommunications will minimize churn by 10–15 percent over the next 18 months. Telecom contact centers can also use predictive analytics based on customer records, technical reports, internal articles of expertise, service tickets and expert advice to speed up call resolution and enhance the customer experience.
Reducing fraud
Telecom fraud is a major reason why the telecom industry lost its income. For example, the annual cost of telecommunications subscription fraud is projected to reach $12B, although others expect that it would be between 3 and 10 percent of the gross revenues of the operators. Telecoms use fraud detection systems based on data mining algorithms to identify fraudulent customers and suspicious activity in near real-time to tackle this issue.
International revenue share fraud (IRSF) is another extremely harmful fraud in the telecoms market. For instance, according to the Communications Fraud Control Association (CFCA), the telecommunications industry has lost about $4B to fraud involving foreign revenue shares (IRSF). IRSF is triggered by artificial traffic inflation or traffic pumping up to the world's premium-rate levels. The fraud is conducted by traffic-generating hackers or fraudsters and PRN (Premium Rate Number) aggregators who acquire a list of fraudulent premium-rate numbers.
Network operations monitoring and management
One of the strategic use cases for mobile network operators (MNOs) is the monitoring and management of network operations. M According to Thractica research, monitoring, and management of network operations is expected to account for 61 percent of AI spending in the sector between 2016 and 2025. And it is only rational because predictive analytics helps to forecast traffic flows and routing peak times, and thus helps to ensure the smooth operation of networks.
Telecoms, for example, can predict the phases of the heaviest usage of the network arising from video sharing and reduce congestion at those times. As a result, telecommunications will reduce their expected capital investment by 15 percent. It also helps to forecast weather effects on the environment (for example, AT&T has developed a special method for assessing climate change). What's more, it goes a long way to directing workflows, managing technician distribution and delivering real-time network alerts.
The future of telecom analytics is with big data
Since a telecom company has to keep up with new technological breakthroughs, stay ahead in one of the most competitive industries in the world, and adhere to a slew of regulations, the function of telecom data analytics is to provide businesses with the easiest way to extract insights from all of their data. Using a solution for telecom data analytics that can help a company obtain deeper insights would produce more revenues.
Every department generates tons of data at a telecommunications and media service provider. Other departments can gain insights into the data from each other without a centralized data analysis. In addition, the data would sometimes vary from those of other departments without a centralized solution, contributing to uncertainty and even frustration.
Free Valuable Insights: Global Telecom Analytics Market to reach a market size of USD 8.7 billion by 2025
All the data departments merge into one unified structure with a centralized data analytics solution. Every department has to provide its insight into the data gleaned, offering a different viewpoint that will lead to a quicker solution. All the departments can benefit from each other, improve performance and increase profitability.
Comments